Federated Learning for Cancer Classification
Marcel Fischer is working on the topics of federated learning and few-shot learning, two machine learning paradigms of high interest in the digital pathology domain.
Federated learning is a machine learning approach that enables multiple devices or organizations to collaboratively train a shared model while keeping their data decentralized. In the context of digital pathology, federated learning can enable multiple institutions to collaboratively develop machine learning models, based on non-shared large data cohorts, especially without compromising sensitive data.
Within the few-shot learning paradigm, the emphasis is on enhancing model performance with minimal labeled data. This is especially valuable in digital pathology, where acquiring and annotating large datasets is often costly and time-intensive.
These broad ideas can be applied to many topics. Marcel is applying them to the assessment of breast cancer tissue and its different stainings that are used in clinical practice.
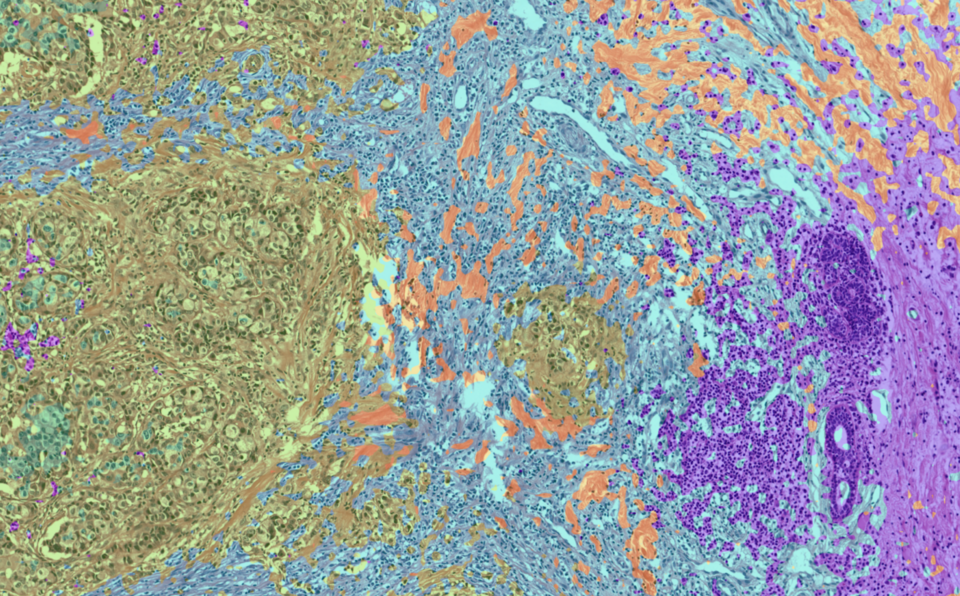
Segmenting breast tissue is a crucial step in breast cancer classification. Federated learning and few-shot learning can enhance this segmentation. Shown here is an unsupervised segmentation of a region from a TCGA-BRCA whole slide image, using F-Seg and UNI. (TCGA: https://portal.gdc.cancer.gov/projects/TCGA-BRCA, F-Seg: https://arxiv.org/abs/2409.05697, UNI: https://arxiv.org/abs/2308.15474, Image: Marcel Fischer)
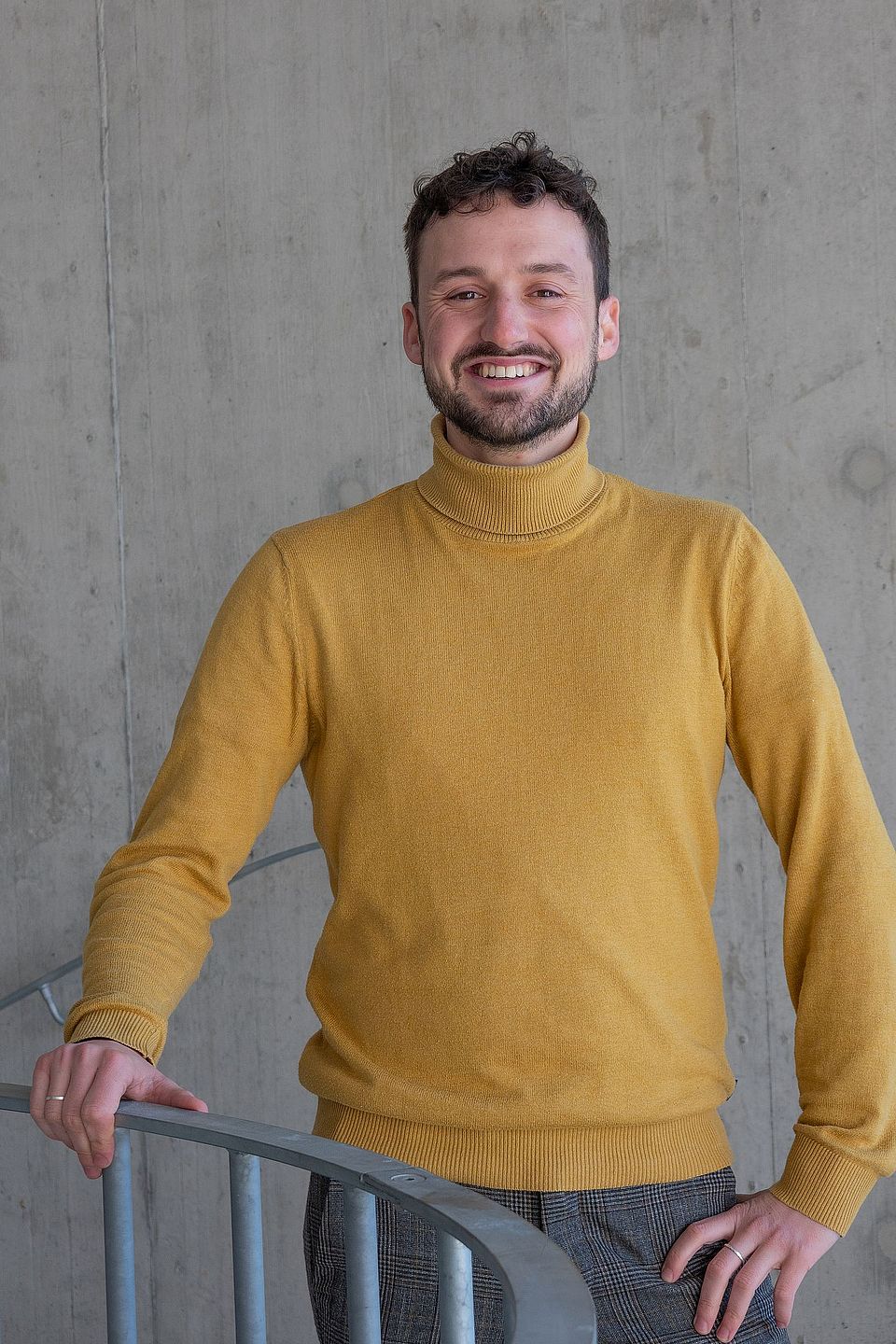
Marcel Fischer