Improving Accessibility to Computational Pathology Models
Within her PhD Lydia is investigating how computational pathology models can be simplified and compressed to enable their usage in low-grade hardware infrastructures, such as consumer-grade computers or smartphones, thereby enabling their usage beyond well-equipped digitized medical facilities to low resource settings.
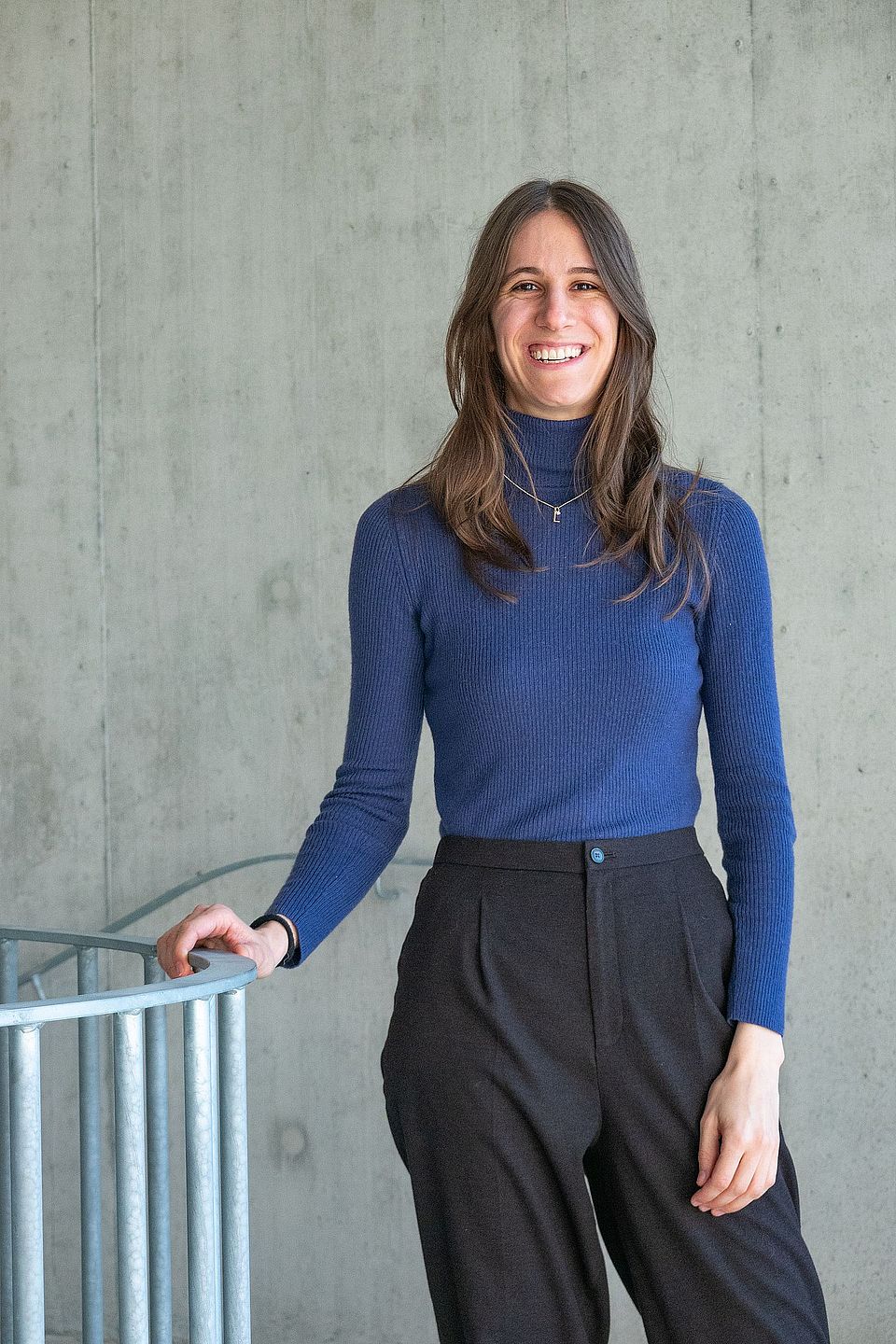
Lydia Schönpflug
Key Papers
Tumour purity assessment with deep learning in colorectal cancer and impact on molecular analysis.
Schoenpflug LA, Chatzipli A, Sirinukunwattana K, Richman S, Blake A, Robineau J, Mertz KD, Verrill C, Leedham SJ, Hardy C, Whalley C, Redmond K, Dunne P, Walker S, Beggs AD, McDermott U, Murray GI, Samuel LM, Seymour M, Tomlinson I, Quirke P; S:CORT consortium; Rittscher J, Maughan T, Domingo E, Koelzer VH.
J Pathol. 2025 Feb;265(2):184-197. doi: 10.1002/path.6376. Epub 2024 Dec 22. PMID: 39710952
A review on federated learning in computational pathology.
Schoenpflug LA, Nie Y, Sheikhzadeh F, Koelzer VH.
Comput Struct Biotechnol J. 2024 Oct 29;23:3938-3945. doi: 10.1016/j.csbj.2024.10.037. eCollection 2024 Dec. PMID: 39582895
SoftCTM: Cell Detection by Soft Instance Segmentation and Consideration of Cell-Tissue Interaction.
Schoenpflug LA, Koelzer VH.
Ahmadi, SA., Pereira, S. (eds) Graphs in Biomedical Image Analysis, and Overlapped Cell on Tissue Dataset for Histopathology. MICCAI 2023. Lecture Notes in Computer Science, vol 14373. Springer, Cham. https://doi.org/10.1007/978-3-031-55088-1_10