How to Codify Complexities of Emergencies?
Overcrowding of Emergency Departments (ED) is of increasing concern. Early detection of patients that need admission might ameliorate medical care. More attention can be given to patients who are severely ill if we detect them early. Patient flow can be improved by early bed reservation, which can be helpful in times of overcrowding. This project aims to predict the need for admission based on as little objective parameters obtained early in the consultation as possible.
Using a dataset obtained from the Basel University Hospital ED during the EMERGE trial, we aim to predict the need for hospital admission. Objective parameters obtained at the initial assessment of the patient (so called "triage"). Predictions whether a patient needs admission - based on age, ambulance use, saturation, heart rate, respiratory rate, temperature systolic and diastolic blood pressure, and pain scale - achieve a mean AUC of 83% and an accuracy of 76%. Adding information obtained later in the process of the ED presentation improves predictions until an AUC of 93% and an accuracy of 85%. For daily use we need predictions as early as possible. Improving these algorithms and making them available to clinicians is part of an ongoing project.
To ensure AI in healthcare is trustworthy, the FUTURE-AI framework was developed as an international consensus guideline. Medical AI tools need to fulfill six guiding principles: Fairness, Universality, Traceability, Usability, Robustness and Explainability. For example, feature importance graphs allow to understand how parameters influence the prediction. This is an important part of the Explainability of a model. Collaboration with clinical partners aims to ensure the Usability of the tool.
Making prediction modes accessible to ED physicians and adapting them in a way they could be used is part of this ongoing project.
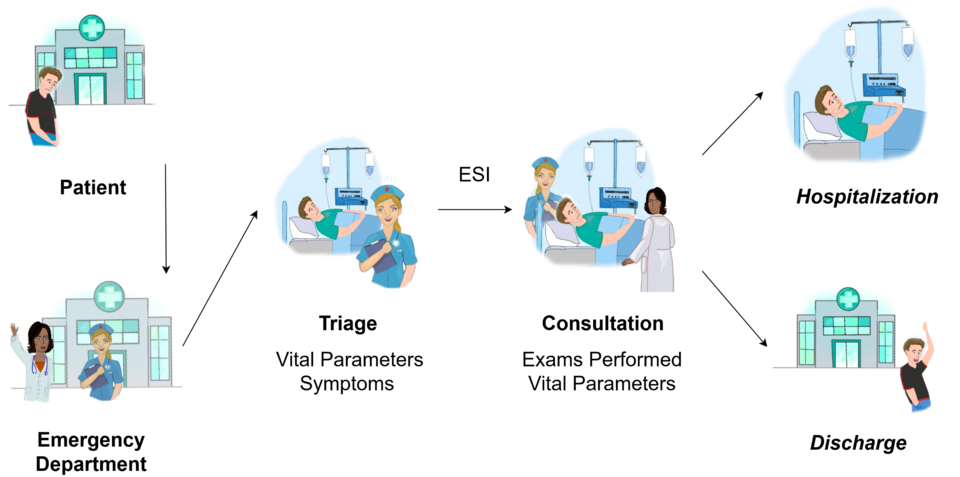
Vital parameters measured when the patient arrives are fed into a model that predicts whether hospitalization is needed as soon as the patient arrives at the ED. Parameters obtained later in the process, as the symptoms of the patient or which exams were performed and vital parameters at the end of the consultation improve the prediction of the model substantially. However, these results are available much later and are thus less helpful when aiming to improve patient flow through the ED.
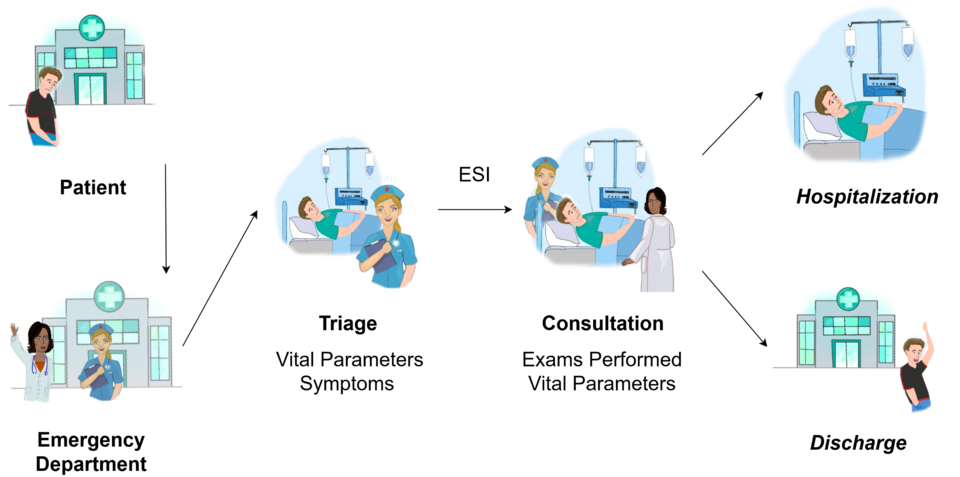
