Automated Image Analysis and Biomarkers detection/segmentation
The diagnosis and monitoring of Multiple Sclerosis progression require the detection and segmentation of various biomarkers, including white matter lesions, contrast-enhancing lesions, paramagnetic rim lesions, cortical lesions, and the central vein sign. Manual detection and segmentation of these biomarkers are time-consuming and prone to significant inter- and intra-rater variability. Deep learning algorithms can support clinicians in this process, but their adoption in clinical workflows depends on their technical performance, reliability, and explainability. Therefore, it is essential to develop robust deep learning methods and thoroughly explore their interpretability.
Examples of the tools developed

From: Contrast-Enhancing Lesion Segmentation in Multiple Sclerosis: A Deep Learning Approach Validated in a Multicentric Cohort. read
The figure illustrates the pipeline for the automatic segmentation of contrast-enhancing lesions. The left section depicts the pre-processing workflow, while the right section highlights the U-Net architecture used to generate the segmentation of contrast-enhanced lesions.
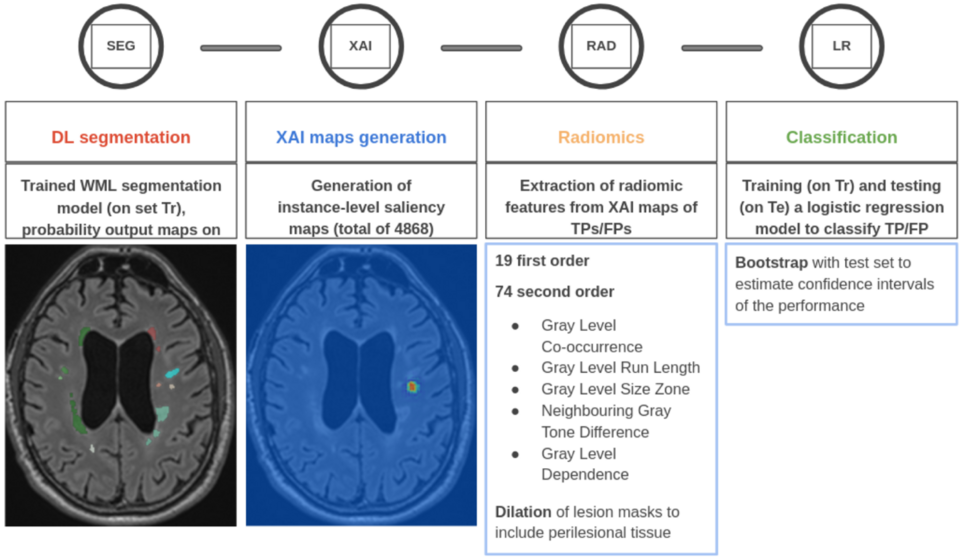
From: Exploiting XAI maps to improve MS lesion segmentation and detection in MRI. read
Overview of the pipeline, from 1) lesion segmentation to 2) the generation of XAI maps, 3) the extraction of radiomic features from XAI maps, and 4) the improvement of lesion classification performances.
- Prof. Dr. Cristina Granziera
- Dr. Po-Jui Lu
- Martina Greselin
- Federico Spagnolo
- Aaisha Bah
- Tim Wölfle
GAMER-MRI in Multiple Sclerosis Identifies the Diffusion-Based Microstructural Measures That Are Most Sensitive to Focal Damage: A Deep-Learning-Based Analysis and Clinico-Biological Validation.
Lu PJ, Barakovic M, Weigel M, Rahmanzadeh R, Galbusera R, Schiavi S, Daducci A, La Rosa F, Bach Cuadra M, Sandkühler R, Kuhle J, Kappos L, Cattin P, Granziera C.
Front Neurosci. 2021 Apr 6;15:647535. doi: 10.3389/fnins.2021.647535. PMID: 33889069; PMCID: PMC8055933. read
GAMER MRI: Gated-attention mechanism ranking of multi-contrast MRI in brain pathology.
Lu PJ, Yoo Y, Rahmanzadeh R, Galbusera R, Weigel M, Ceccaldi P, Nguyen TD, Spincemaille P, Wang Y, Daducci A, La Rosa F, Bach Cuadra M, Sandkühler R, Nael K, Doshi A, Fayad ZA, Kuhle J, Kappos L, Odry B, Cattin P, Gibson E, Granziera C.
Neuroimage Clin. 2021;29:102522. doi: 10.1016/j.nicl.2020.102522. Epub 2020 Dec 3. PMID: 33360973; PMCID: PMC7773673. read
Contrast-Enhancing Lesion Segmentation in Multiple Sclerosis: A Deep Learning Approach Validated in a Multicentric Cohort.
Greselin M, Lu PJ, Melie-Garcia L, Ocampo-Pineda M, Galbusera R, Cagol A, Weigel M, de Oliveira Siebenborn N, Ruberte E, Benkert P, Müller S, Finkener S, Vehoff J, Disanto G, Findling O, Chan A, Salmen A, Pot C, Bridel C, Zecca C, Derfuss T, Lieb JM, Diepers M, Wagner F, Vargas MI, Pasquier RD, Lalive PH, Pravatà E, Weber J, Gobbi C, Leppert D, Kim OC, Cattin PC, Hoepner R, Roth P, Kappos L, Kuhle J, Granziera C.
Bioengineering (Basel). 2024 Aug 22;11(8):858. doi: 10.3390/bioengineering11080858. PMID: 39199815; PMCID: PMC11351944. read
Instance-level quantitative saliency in multiple sclerosis lesion segmentation
Spagnolo F, Molchanova N, SchaerR, Bach Cuadra M, Ocampo Pineda M, Melie-Garcia L, Granziera C, Andrearczyk V, Depeursinge A
arXiv:2406.09335v2 [eess.IV] read
Exploiting XAI maps to improve MS lesion segmentation and detection in MRI
Spagnolo F, Molchanova N, Ocampo Pineda M, Melie-Garcia L, Bach Cuadra M, Granziera C, Andrearczyk V, Depeursinge A
bioRxiv 2024.08.29.610090; doi: https://doi.org/10.1101/2024.08.29.610090 read
How far MS lesion detection and segmentation are integrated into the clinical workflow? A systematic review
Spagnolo F, Depeursinge A, Schädelin S, Akbulut A, Müller H, Barakovic M, Melie-Garcia L, Bach Cuadra M, Granziera C.
Neuroimage Clin. 2023;39:103491. doi: 10.1016/j.nicl.2023.103491. Epub 2023 Aug 12. read